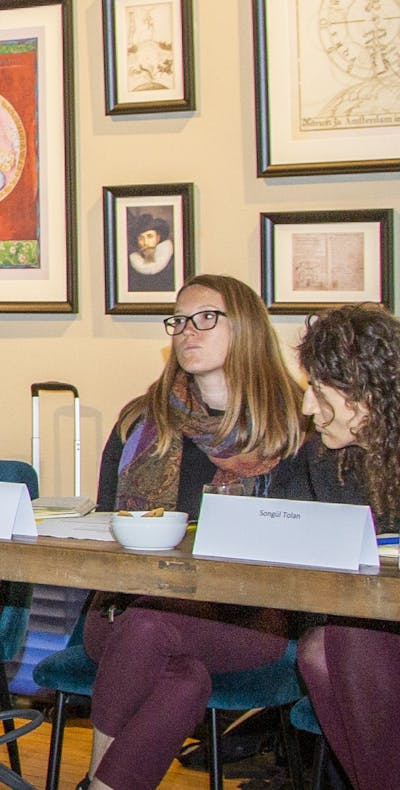
PHRP Expert Meeting on Predictive Policing
On 20.-21. May 2019, the Police and Human Right Programme (PHRP) of Amnesty International Netherlands held an expert meeting on predictive policing. The meeting brought together representatives of civil society organisations, law enforcement agencies, international institutions as well as researchers from the academic and the business sector.
The increasing use of algorithms in policing work raises important questions both in terms of their effectiveness to actually improve policing as well as in terms of their human rights impact. Data protection and right to privacy, freedom from discrimination, fair trial guarantees, right to liberty and security, right to an effective remedy are just a few of the human rights issues at stake.
Place-oriented predictive policing
In place-oriented predictive policing a wide range of data are used with a view to predict areas where crimes are likely to be committed in the near future. The discussions during the expert meeting revealed the following challenges and problems:
- Data quality and possible bias: Input data used to train the algorithmic model often do not reflect the complete reality. They may be incomplete, outdated or the result of selective, if not biased policing approaches in the past, e.g. if police previously focused particularly on a given community or category of people. Outputs therefore need to be reviewed with care: Where there are indications of bias, it can be very problematic to use them as a basis for decision making, since this may lead to, or exacerbate existing, discriminatory police approaches. Instead of taking such outputs for granted and using them as a decision-making tool, they might better be used as a diagnostic tool, seeking to identify the reasons for such an output, e.g. why certain groups or places appear in the output as having greater involvement in crime. This might help police to improve their approach and their relationship with certain communities as well as to address underlying causes of crime rather than simply to increase policing of certain areas or communities.
- Inaccuracy and risk of bias in the designed algorithmic model: Besides the underlying data, the design of the model can be biased itself or it can use statistically irrelevant features that will lead to irrelevant correlations and outputs. The more features are used, the greater will be the risk of irrelevant correlations. Furthermore, self-learning systems bear the risks of exacerbating differences overtime with the continuous input over time. This risk can furthermore increase through feedback-loops when policing approaches may change the situation on the ground which feed back into the system (e.g. more crime is detected in an area where police are increasingly patrolling, while others remain undetected in under-policed areas). Enhanced testing of models through variations of input data in order to identify potential bias as well as reinforced learning (counter-balancing feedback loops) were mentioned as possible remedies. These, however, have not yet reached the stage of implementation in practice. Again, this may lead to the conclusion that output of automated systems might rather be used as a diagnostic tool to identify causes for differences between groups rather than as a decision-making tool that can then lead to possible discrimination of one group against others.
- Measuring the effectiveness in the prevention of crime was found to be very difficult. If police decide to increase patrolling as a result of a prediction, it will be virtually impossible to ascertain the accuracy of the prediction, if no crime is taking place – as a result of the patrolling that achieved the desired deterring effect or because the prediction was simply wrong. In addition, cyclical fluctuations of crime as well as the possible impact of other policing measures or crime-relevant factors can influence crime statistics. Furthermore, other elements, such as the impact of predictive policing methods on communities and police-community relationship should be included in the evaluation of the usefulness of these systems as well. In view of these aspects, predictive policing methods should only be introduced based on a clearly defined operational goal that takes all these questions into account.
- While predictive policing methods are often introduced with a view to compensate a lack of resources, it was highlighted that the costs of predictive policing methods (design, testing and evaluating the system, as well as implementing policing approaches accordingly) tend to be underestimated.
Person-oriented predictive policing
In person-oriented predictive policing, data are used to predict the likelihood for an individual to commit crime, e.g. in order to assess risk of recidivism or the potential of a person to be involved a violent act. The discussions revealed that some of the problems already mentioned above become exacerbated when it comes to the way how the use of algorithms can affect the lives of individuals on whom these algorithms are applied:
- Discrimination due to biased input data or bias in the design of the model is particularly problematic when decisions are applied at an individual level. The mere fact of a person belonging to a specific group may then lead to decisions that heavily affect the human rights of this person in a discriminating manner. Even when specific sensitive features such as ethnicity, nationality etc. are not explicitly included in the model, correlations with other features may in the end lead to these aspects being considered by the system and result in a biased output. Correcting such a bias is difficult, since it requires the certainty that an output is the result of bias and not reflecting the reality.
- Since person-oriented predictive policing can have considerable impact on a person’s life (e.g. the decision to release a person or not, to arrest a person or not), the problem of false positives becomes particularly relevant. Often these systems are presented as being highly accurate, but this is in many cases only correct in relation to the true positives, e.g. persons being correctly identified as presenting a high risk, since they indeed committed a/another crime. The high percentage of persons being wrongly classified high risk (the false positives) is usually overlooked, but it is in terms of human rights impact the most serious problem in the use of algorithmic models: decisions severely affecting the lives of people are taken based on a wrong assumption of them presenting a danger. Here, the principles of proportionality and “guilt proven beyond reasonable doubt” would need to be given greater consideration.
- Despite the risks of false positives, the likelihood of an output being decisive for a decision taken in relation to an individual is very high, since the responsibility to take a “wrong” decision would considerably increase, e.g. when a judge decides to “overrule” the output recommendation of an automated system.
Accountability
Predictive policing methods present particular challenges in terms of accountability:
- Participants agreed that there is a need to establish clear rules for the development and use of such systems and that these processes should also be governed by an ethical framework to prevent discrimination and excessive impact on human rights.
- This, however, was found to be problematic due to the technical complexity of the systems that are difficult to assess. This difficulty is further exacerbated in case of self-learning systems which even the designer will become unable to evaluate at a certain point. In addition, the proper assessment of how (good or bad) the system works is often hampered due to a lack of transparency when private companies are involved.
- Challenging decisions taken based on an automated process is furthermore difficult, when the degree of influence of the automated output on a human decision is unclear, e.g. whether the decision of a judge to deny bail was taken in a reflected manner or simply by following the output score. And a mathematically correctly calculated average score given to an individual is virtually impossible to challenge as such. The possibilities of the affected person to obtain a review are then actually limited to challenging the more principled question of being given such a score without the possibility to have his or her personal situation being assessed, as well as to have the decision reviewed that was taken in the end (despite the score given by the system).
- Finally, it was agreed during the meeting that there is an urgent need for those involved in ensuring proper accountability for the decision taken on the basis of an algorithm, in particular judges, to have the knowledge and expertise about how results are obtained within an automated system (including the possible weaknesses).
Conclusion and outlook
Further research, reflection and discussion is needed in this highly complex area in relation to the data quality, the accuracy of the models used, measuring the effectiveness of crime prevention through the use of algorithms, the prevention of discriminatory, unnecessary and/or disproportionate impact on human rights, as well as ensuring sufficient and effective transparency and accountability.
Anybody interested in exchange with PHRP on these questions is warmly welcomed to contact us under: phrp@amnesty.nl